Reshaping Compute
An API to improve the accuracy, data efficiency and power consumption of neural networks
WHO WE ARE
A deep-tech company.
We are working at building a new computational paradigm to improve the accuracy, data efficiency and power consumption of neural networks.
A leading team of deep learning experts
Our team is composed of experienced deep learning and HPC researchers and developers with publications in leading scientific journals and conferences.
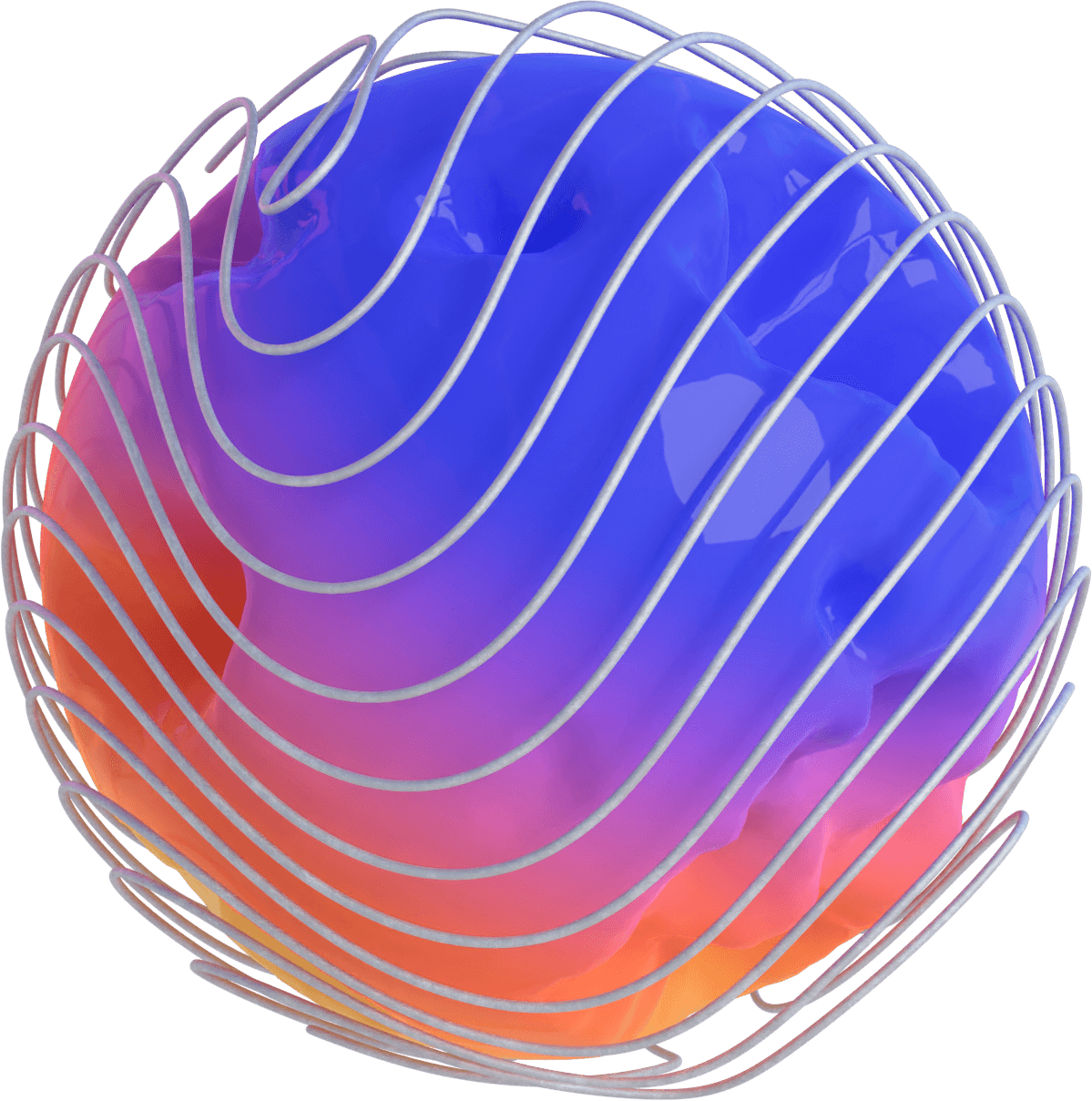
WHAT OUR MISSION IS
Current technology can not keep up with AI’s growing demand for computing.
It is time for a new computational paradigm.
- The way computers are designed can not sustain the scale of computation necessary to keep us all connected.
- We are rethinking the mathematical foundations behind classical computation and developing new ways of computations more efficient at extracting information
from data. - By going beyond linear algebra we want to unlock new technological breakthroughs that will achieve unprecedented results in both software and hardware.
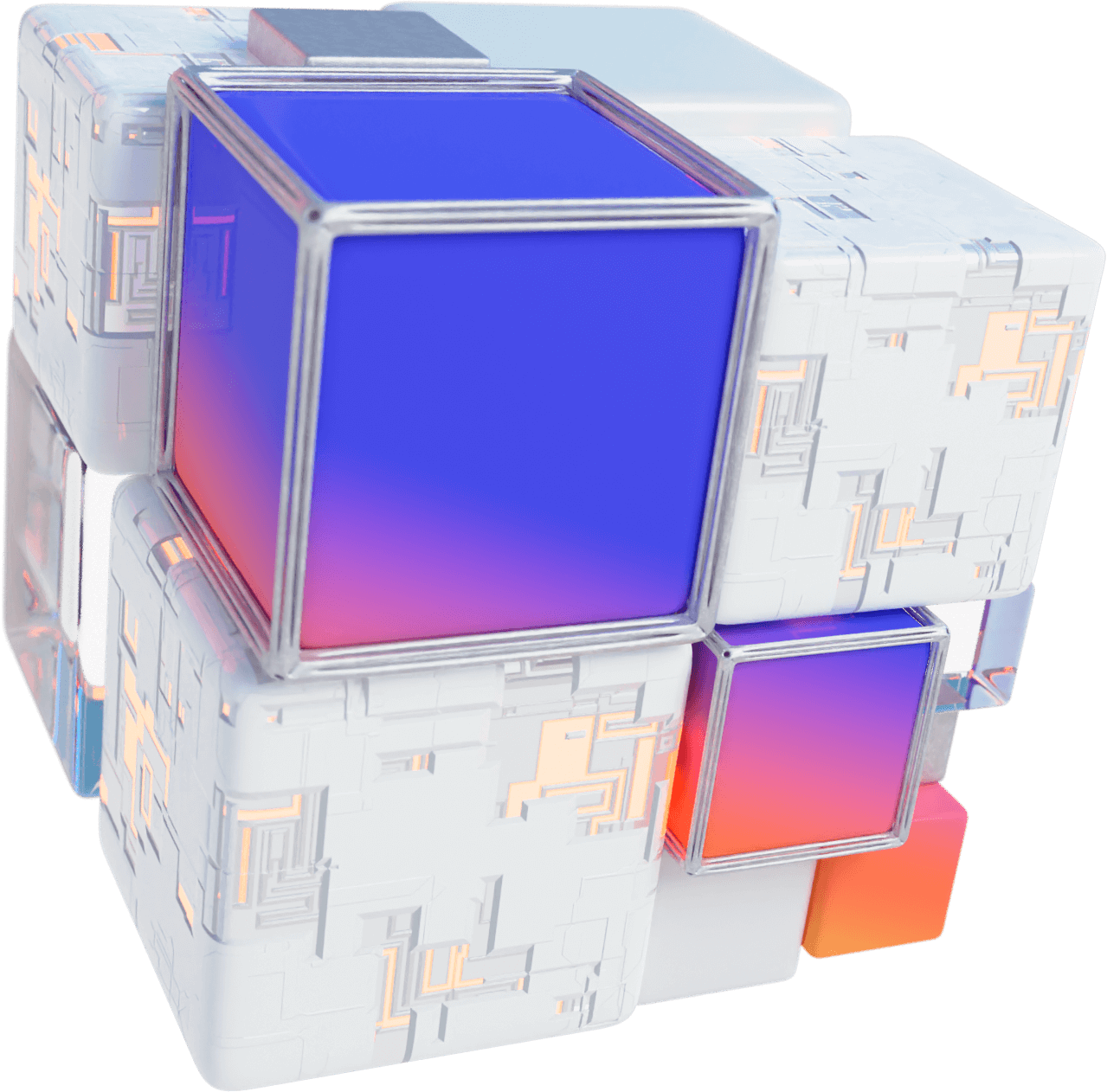
WHAT WE ARE SOLVING
Deep Learning is not computationally expensive by accident, but by design.
- Deep Learning is data hungry +
- Deep Learning is computationally expensive +
- Deep learning is hard to deploy on edge devices +
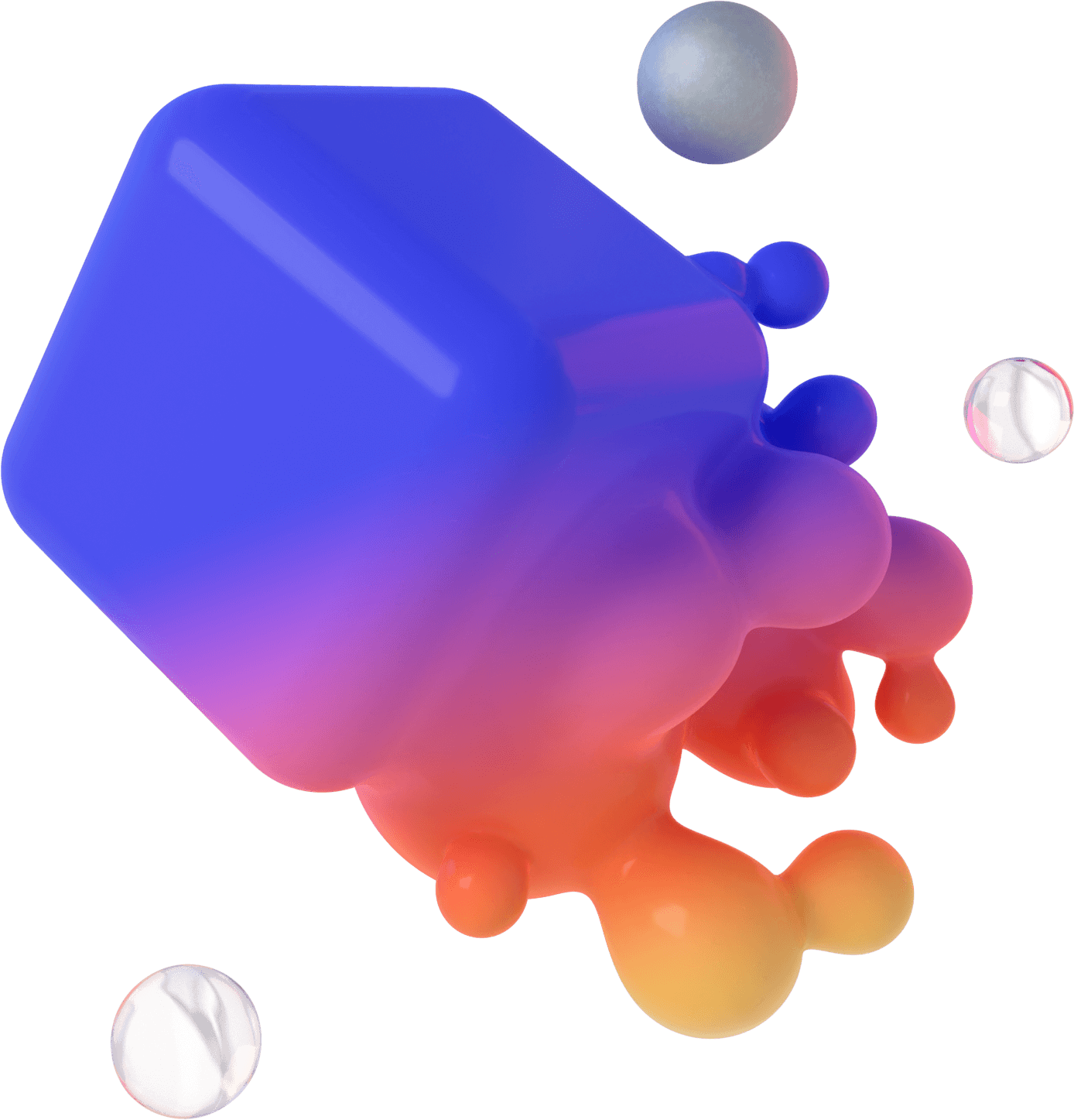
WHAT OUR PRODUCT IS
Boost the performance of your deep learning networks with our intuitive and easy to use API for computer vision.
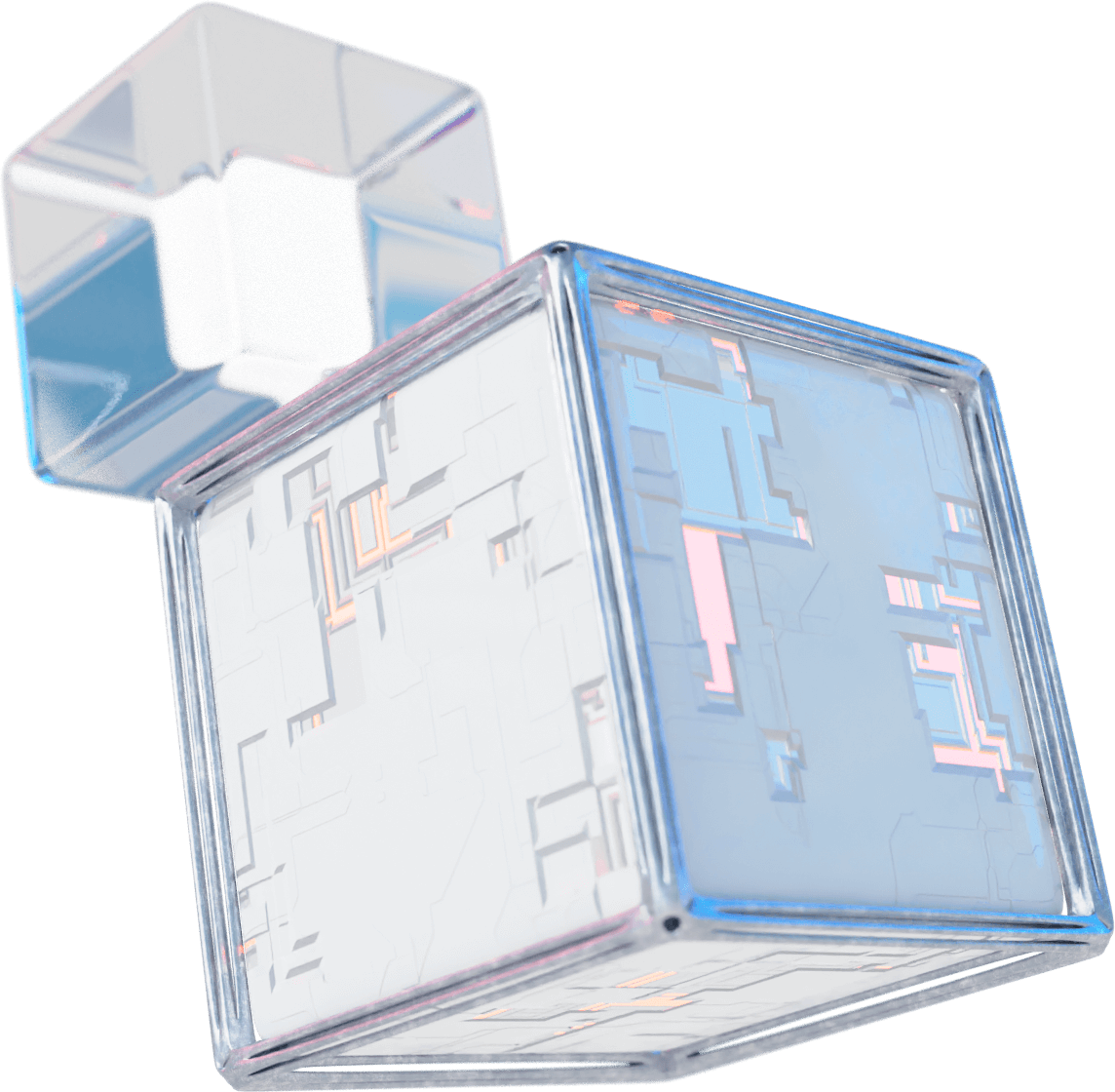
Build models with less data
Achieve your accuracy needs with less data. Slash the cost of new data acquisition and labelling to create faster state-of-the art solutions at better price.
Increase your model accuracy
Upstride’s technology allows you toretrain your model with an increase in accuracy with the same dataset. Go to production quicker and explore new use cases.
Compress your model size
Upstride’s technology allows it to build smaller models with the same performance. Deploy on edge devices and run models locally with less power.